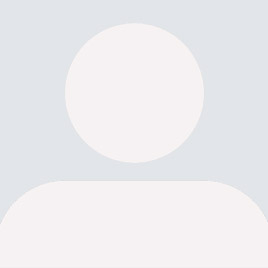
Dr. Shreejith Shanker
Assistant Professor, Electronic & Elect. Engineering
Email shankers@tcd.ie Phone3531896 1609https://shreejithshanker.github.ioBiography
Dr. Shanker is an Assistant Professor at the Department of Electronic & Electrical Engineering at Trinity College Dublin, The University of Dublin, Ireland, since April 2019. He graduated with a Bachelors degree in Electronics & Communication Engineering from the former the University of Kerala {currently called APJ Abdul Kalam Technological University} in 2006 and a PhD degree from Nanyang Technological University and Technical University of Munich in 2016. From 2015 to 2016, he was a post-doctoral research fellow at the Hardware and Embedded Systems Lab, Nanyang Technological University, Singapore where he was working on cognitive radio architectures and techniques for commercial and aeronautical communication systems. He took up the role of Teaching Fellow at the School of Engineering, The University of Warwick, UK in 2017, where he continued his research on in-network computation and accelerators, while primarily focussing on delivering modules on Computer Architecture and Programming to the undergraduate cohort. He briefly took up the role of Research Fellow at the Electrification Suite and Test Lab , TUM CREATE Ltd, Singapore, exploring research ideas on decentralised compute systems in smart energy systems and power grids before taking up the role of Assistant Professor at Trinity College Dublin, Ireland. His current research explores reconfigurable architectures and frameworks for distributed accelerators that are tightly coupled to the network fabric, with application to autonomous systems, media processing and communication networks. He started his professional career in 2006 as a Design Engineer at Processor Systems India where he was involved in design and verification of high-speed custom logic for network switches and compute accelerators. Later, he joined the Vikram Sarabhai Space Centre, one of the premier research centres under the Indian Space Research Organisation as a Scientist working on design of real-time, mission critical subsystems for launch vehicles and satellite systems.
Publications and Further Research Outputs
- Shreejith, Shanker and Vipin, Kizhepatt and Fahmy, Suhaib A and Lukasiewycz, Martin, An approach for redundancy in FlexRay networks using FPGA partial reconfiguration, 2013 Design, Automation \& Test in Europe Conference \& Exhibition (DATE), 2013, pp721--724Conference Paper, 2013
- Shreejith, Shanker and Fahmy, Suhaib A and Lukasiewycz, Martin, Reconfigurable Computing in Next Generation Automotive Networks, Embedded Systems Letters, 5, (1), 2013, p12--15Journal Article, 2013
- Lukasiewycz, Martin and Steinhorst, Sebastian and Andalam, Sidharta and Sagstetter, Florian and Waszecki, Peter and Chang, Wanli and Kauer, Matthias and Mundhenk, Philipp and Shanker, Shreejith and Fahmy, Suhaib A and others, System architecture and software design for electric vehicles, Proceedings of the 50th Annual Design Automation Conference, 2013, pp1--6Conference Paper, 2013
- Shreejith, Shanker and Fahmy, Suhaib A and Lukasiewycz, Martin, Accelerating Validation of Time-Triggered Automotive Systems on FPGAs, International Conference on Field Programmable Technology (ICFPT), 2013Conference Paper, 2013
- Vipin, Kizheppatt and Shreejith, Shanker and Gunasekera, Dulitha and Fahmy, Suhaib A and Kapre, Nachiket, System-Level FPGA Device Driver with High-Level Synthesis Support, International Conference on Field Programmable Technology (ICFPT), 2013Conference Paper, 2013
- Shreejith, Shanker and Fahmy, Suhaib A, Enhancing Communication On Automotive Networks Using Data Layer Extensions, International Conference on Field Programmable Technology (ICFPT), 2013Conference Paper, 2013
- Shreejith, S and Fahmy, S. A, Extensible FlexRay Communication Controller for FPGA-Based Automotive Systems, Transactions on Vehicular Technology, 64, (2), 2015, p453--465Journal Article, 2015
- Vipin, Kizheppatt and Shreejith, Shanker and Fahmy, Suhaib A and Easwaran, Arvind, Mapping time-critical safety-critical cyber physical systems to hybrid FPGAs, IEEE International Conference on Cyber-Physical Systems, Networks, and Applications, 2014, pp31--36Conference Paper, 2014
- Lukasiewycz, Martin and Shreejith, Shanker and Fahmy, Suhaib A, System simulation and optimization using reconfigurable hardware, International Symposium on Integrated Circuits (ISIC), 2014, pp468--471Conference Paper, 2014
- Shreejith, Shanker and Fahmy, Suhaib A, Zero latency encryption with FPGAs for secure time-triggered automotive networks, International Conference on Field-Programmable Technology (FPT), 2014, pp256--259Conference Paper, 2014
- Shreejith, Shanker and Banarjee, Bhaskar and Vipin, Kizheppatt and Fahmy, Suhaib A, Dynamic Cognitive Radios on the Xilinx Zynq Hybrid FPGA, International Conference on Cognitive Radio Oriented Wireless Networks (CROWNCOM), Doha, Qatar, 2015Conference Paper, 2015
- Shreejith, Shanker and Fahmy, Suhaib A., Security Aware Network Controllers for Next Generation Automotive Embedded Systems, Proceedings of the 52nd Design Automation Conference (DAC), 2015Conference Paper, 2015
- Ambede, A. and Shreejith, S. and Vinod, A.P. and Fahmy, S.A., Design and Realization of Variable Digital Filters for Software Defined Radio Channelizers using Improved Coefficient Decimation Method, IEEE Transactions on Circuits and Systems II:, 2015Journal Article, 2015
- Fahmy, Suhaib A and Vipin, Kizheppatt and Shreejith, Shanker, Virtualized FPGA accelerators for efficient cloud computing, 2015 IEEE 7th International Conference on Cloud Computing Technology and Science (CloudCom), 2015, pp430--435Conference Paper, 2015
- Shreejith, Shanker and Anshuman, Bezborah and Fahmy, Suhaib A, Accelerated artificial neural networks on FPGA for fault detection in automotive systems, 2016 Design, Automation \& Test in Europe Conference \& Exhibition (DATE), 2016, pp37--42Conference Paper, 2016
- Shreejith, Shanker and Ambede, Abhishek and Vinod, AP and Fahmy, Suhaib A, A power and time efficient radio architecture for LDACS1 air-to-ground communication, 2016 IEEE/AIAA 35th Digital Avionics Systems Conference (DASC), 2016, pp1--6Conference Paper, 2016
- Shanker, Shreejith, Enhancing Automotive Embedded Systems with FPGAs, 2016Thesis, 2016
- Warrier, Rakesh and Shreejith, Shanker and Zhang, Wei and Vun, Chan Hua and Fahmy, Suhaib A, Fracturable DSP block for multi-context reconfigurable architectures, Circuits, Systems, and Signal Processing, 36, (7), 2017, p3020--3033Journal Article, 2017
- Shreejith, Shanker and Mundhenk, Philipp and Ettner, Andreas and Fahmy, Suhaib A and Steinhorst, Sebastian and Lukasiewycz, Martin and Chakraborty, Samarjit, VEGa: A high performance vehicular Ethernet gateway on hybrid FPGA, IEEE Transactions on Computers, 66, (10), 2017, p1790--1803Journal Article, 2017, TARA - Full Text
- Ambede, A. and Shreejith, S. and Vinod, A.P, Efficient FPGA Implementation of a Variable Digital Filter based Spectrum Sensing Scheme for Cognitive IoT Systems, Global IoT Summit, 2017Conference Paper, 2017
- Shreejith, Shanker and Mathew, Libin K and Prasad, Vinod A and Fahmy, Suhaib A, Efficient spectrum sensing for aeronautical LDACS using low-power correlators, IEEE Transactions on Very Large Scale Integration (VLSI) Systems, 26, (6), 2018, p1183--1191Journal Article, 2018
- Shreejith, Shanker and Fahmy, Suhaib A, Smart network interfaces for advanced automotive applications, IEEE Micro, 38, (2), 2018, p72--80Journal Article, 2018
- Shreejith, Shanker and Cooke, Ryan A and Fahmy, Suhaib A, A smart network interface approach for distributed applications on Xilinx Zynq SoCs, 2018 28th International Conference on Field Programmable Logic and Applications (FPL), 2018, pp186--1864Conference Paper, 2018
- Bucknall, Alex R and Shreejith, Shanker and Fahmy, Suhaib A, Network Enabled Partial Reconfiguration for Distributed FPGA Edge Acceleration, International Conference on Field Programmable Technology (ICFPT), 2019Conference Paper, 2019
- Mathew, Libin K and Shanker, Shreejith and Vinod, AP and Madhukumar, AS, An Adaptive Energy Detection Scheme with Real-Time Noise Variance Estimation, Circuits, Systems, and Signal Processing, 2019, p1--25Journal Article, 2019
- Shivaraman, Nitin and Ramanathan, Saravanan and Shanker, Shreejith and Easwaran, Arvind and Steinhorst, Sebastian, DeCoRIC: Decentralized Connected Resilient IoT Clustering, International Conference on Computer Communications and Networks (ICCCN), USA, 2020Conference Paper, 2020, TARA - Full Text
- Mathew, Libin K and Shanker, Shreejith and Vinod, AP and Madhukumar, AS, A Power-Efficient Spectrum-Sensing Scheme Using 1-Bit Quantizer and Modified Filter Banks, IEEE Transactions on Very Large Scale Integration (VLSI) Systems, 28, (9), 2020, p2074--2078Journal Article, 2020
- Emanuel Regnath, Nitin Shivaraman, Shanker Shreejith, Arvind Easwaran, Sebastian Steinhorst, Blockchain, what time is it? Trustless Datetime Synchronization for IoT, International Conference on Omni-layer Intelligent Systems (COINS), Barcelona, Spain, September, 2020, 2020Conference Paper, 2020, TARA - Full Text
- Alex R Bucknall, Shanker Shreejith, Suhaib A Fahmy, Build automation and runtime abstraction for partial reconfiguration on Xilinx Zynq Ultrascale+, International Conference on Field-Programmable Technology, December, 2020, 2020Conference Paper, 2020, TARA - Full Text
- Thinh H. Pham, Shanker Shreejith, Sebastian Steinhorst, Suhaib A. Fahmy, Samarjit Chakraborty, Heterogeneous Communication Virtualization for Distributed Embedded Applications, Euromicro Conference on Digital System Design (DSD), Palero, Italy, September, 2021, IEEE, 2021, pp10.1109/DSD53832.2021.00047Conference Paper, 2021, TARA - Full Text
- Shashwat Khandelwal, Eashan Wadhwa, Shreejith Shanker, Deep Learning-based embedded Intrusion Detection Systems for CAN bus in Automotive Networks, 33rd IEEE International Conference on Application-specific Systems, Architectures and Processors, Gothenburg, Sweden, July, 2022, IEEE, 2022Conference Paper, 2022, TARA - Full Text
- Eashan Wadhwa, Shashwat Khandelwal, Shreejith Shanker, IMEC: A Memory-Efficient Convolution Algorithm For Quantised Neural Network Accelerators, 33rd IEEE International Conference on Application-specific Systems, Architectures and Processors, Gothenburg, Sweden, July 2022, IEEE, 2022Conference Paper, 2022, TARA - Full Text
- Daniel Flood, Neethu Robinson and Shanker Shreejith, FPGA-based Deep-Learning Accelerators for Energy Efficient Motor Imagery EEG classification, 2022 IEEE International Conference on Omni-layer Intelligent Systems (COINS) , Barcelona, Spain, August 1-3, 2022Conference Paper, 2022, TARA - Full Text
- Shashwat Khandelwal and Shanker Shreejith, A Lightweight Multi-Attack CAN Intrusion Detection System on Hybrid FPGAs, International Conference on Field Programmable Logic and Applications (FPL), Belfast, UK, Aug 29-31, 2022Conference Paper, 2022, TARA - Full Text
- Shashwat Khandelwal, Shanker Shreejith, A Lightweight FPGA-based IDS-ECU Architecture for Automotive CAN, International Conference on Field Programmable Technology , Hong Kong SAR, China, IEEE, 2022Conference Paper, 2022, TARA - Full Text
- Shashwat Khandelwal, Anneliese Walsh, Shanker Shreejith, Quantised Neural Network Accelerators for Low-Power IDS in Automotive Networks, Design Automation and Test in Europe, Antwerp, Belgium, 17 - 19 April, 2023, 2023Conference Paper, 2023
- Shashwat Khandelwal & Shanker Shreejith, Exploring Highly Quantised Neural Networks for Intrusion Detection in Automotive CAN, International Conference on Field Programmable Logic and Applications (FPL), September, 2023, 2023Conference Paper, 2023, TARA - Full Text
- Shashwat Khandelwal & Shanker Shreejith, Real-time zero-day Intrusion Detection System for Automotive Controller Area Network on FPGAs, International Conference on Application-specific Systems, Architectures and Processors, Portugal, July 2023, 2023Conference Paper, 2023, TARA - Full Text
- Boyle, Jason and Shanker, Shreejith, A case for FPGA-based accelerators for energy-efficient motion picture video processing, Applications of Digital Image Processing XLVI, San Diego, August, 2023, edited by SPIE , SPIE, 2023Conference Paper, 2023, TARA - Full Text
- Emmet Murphy, Shashwat Khandelwal, Shanker Shreejith, Custom precision accelerators for energy-efficient image-to-image transformations in motion picture workflows, Applications of Digital Image Processing XLV., San Diego, USA, August, 2023, SPIE, 2023Conference Paper, 2023, TARA - Full Text
- Abhishek Duttagupta, Jin Zhao, Shanker Shreejith, Exploring Lightweight Federated Learning for Distributed Load Forecasting, IEEE SmartGridComm 2023 Conference, Glasgow, UK, 31/10/2023, 2023Conference Paper, 2023, TARA - Full Text
Research Expertise
My research area focuses on building computer architectures that enable unique ways for improving compute efficiency, network performance and provide reactive capabilities to adapt to changing environments, through seamless interaction of software and hardware. My research applies this approach to tailor compute, network and deep-learning architectures to enable data-driven real-time reactive solutions in different domains such as automotive embedded systems, cognitive radio systems, and biomedical systems. A key enabler for his research is fully programmable platforms (or reconfigurable hardware), which enables both the software and the underlying hardware to be adapted to the compute requirements and specifications, either statically (i.e., at design time) or dynamically (i.e., at run-time). My current research direction focuses on enabling energy-efficient ways to perform compute-intensive data-driven tasks such as (edge-) cloud analytics or deep learning inference by optimising different components of the system - from low-level computational building blocks that enable efficient offload of compute-intensive tasks, to the software APIs that interface with the accelerators, and compiler tools to automate the development and deployment of these solutions. This combined strategy enables right-sizing of operations, interconnection, storage and data movement, which are critical components in reducing the energy footprint of such data-intensive tasks. In our current research, we are exploring three key application areas - secure connected automotive systems, bio-information systems for smart health, and high-performance video streaming/processing pipelines for visual algorithms in cloud/on-premise. Additionally, we also explore decentralisation of these compute tasks and consensus schemes to enable novel applications that preserve privacy in sensitive data-driven tasks to augment our approach.
-
TitleResource-efficient Deep-Learning for Microwave Breast Image ReconstructionSummaryIn this project, the use of machine learning for microwave breast image reconstruction is to be explored. Specifically, this project will examine two key questions: firstly, the feasibility of directly learning a microwave breast imaging reconstruction algorithm that is generalisable and stable; secondly, the development of a custom digital design for a resource-efficient implementation of a fully learned reconstruction algorithm. Together, these questions help assess if recent developments in medical imaging could help accelerate the translation of highly efficient microwave breast imaging to clinical useFunding AgencyTCD
-
TitleHA4DML: HETEROGENEOUS ARCHITECTURES FOR DISTRIBUTED MACHINE LEARNINGSummaryIn the proposed project, we aim to investigate the compute architecture, algorithmic techniques and tool flow for deploying decentralised-federated learning at or close to the source of the data in a cyber-physical system (CPS). In many practical Internet of Things (IoT) and cyber-physical applications, the sensors and processing elements close to sensors have restricted compute capabilities locally to consume as little energy as possible, particularly on battery-operated systems. In such cases, computation is carried out in a centralised node that connects to the different sensors to acquire their observations. Centralised processing results in a single point of failure, reduced observability and controllability, as well as increased reaction times, which are crucial factors in critical CPSs. Decentralisation allows small fragments of computing to be performed close to the source, improving the overall performance and reliability of the system. Decentralisation also works well in the case of approximate computing and machine learning models, where models can be trained and optimised for a subset of sensors much more efficiently to achieve highly accurate predictions. Federated learning builds on this premise to create a hierarchy of models, where at each layer, the model is fine-tuned to its observations, while also contributing to the improved global predictions.Funding AgencyIrish Research Council
-
TitleLight-weight Distributed Intrusion Detection for Automotive NetworksSummaryModern vehicles are complex machines driven by electronics, sensors and software. Increasingly, they have become targets for malicious code injections and attacks. Connectivity within a vehicle, predominantly based on legacy networks like CAN, has not evolved to cater to such attacks, while complex rule-based attack detection and prevention systems are inefficient (cost, energy etc). Deep Learning (DL) based solution offers a promising route - however, the computational complexity of DL networks needs to be addressed. This project explores a system-level architecture that enables DL-based intrusion detection to be integrated seamlessly into existing automotive networks. The key challenges we are trying to solve is the low-latency requirements for line-rate detection and the energy overheads of DL-based methods.Funding AgencyTCD InternalDate From01/04/2021
-
TitleA Decentralised Federated Learning Framework for Smart Energy System in IrelandSummaryIn this project, we wanted to investigate the possibility of decentralised-federated learning in the context of energy systems to enable real-time monitoring and management, prediction of load demands and estimation of stability of the network at a level of granularity finer than what is possible through existing techniques based on information and communication technologies (ICT) like smart-meter coordinated (smart) grids. The key challenges we were trying to address is the privacy issues associated with monitoring and analysing energy consumption data as well as the algorithmic and system architecture components for integrating the proposed approach.Funding AgencyScience Foundation Ireland
-
TitleDISCLOSE: Distributed Sensing and Collaborative Optimisation for Smart Energy-efficient buildingsSummaryThe scientific aim of the project is to develop a framework for distributed electricity consumption monitoring and active load management at a granular level within a network of energy- consuming devices. The project will enable, in real-time, the interaction between devices to aggregate overall energy consumption, determine control actions and deploy them in a distributed and cooperative manner.Funding AgencySEAI
-
TitleMUSICAL: MUltiSensing with photonic Integrated Circuits for Accurate Live monitoringSummaryDriven by the need for pervasive data acquisition in an increasingly connected world, often in the Internet-of-things (IoT) scenarios. Hence, the availability of small, inexpensive, accurate, easy to use and energy efficient sensing systems has a potential to deliver significant economic, environmental and societal impact. Photonic sensors are an ideal foil for such systems as they lend themselves to precise and highly sensitive analysis in a wide range of applications (gas, vibration, temperature, strain etc.). However, most of the proof-of-concept experiments are performed in controlled laboratory environments. More importantly, they often rely on benchtop-type measurement equipment, such as highly stable tunable lasers or high-resolution spectrometers for operation and readout. Photonic sensors, through integration, are amenable to miniaturization and cost-efficient mass production thereby enabling innovative and high functionality components to be multiplexed together on a single photonic chip. Thus, the goal of MUSICAL is to develop a fully integrated, highly accurate and energy efficient modular multi-sensing photonic system that can be used in all the above-mentioned applications operated under real-world constraints. Its modular approach will enable flexibility and adaptability of the system, allowing it to be optimised for the specific requirements of an individual application. The development of the hardware platform will be accompanied by an investigation into efficient and flexible processing algorithms of the sensory data. This will further contribute to the flexibility and energy efficiency of the entire multi-sensing solution. During the project, the PIC platform will be designed, fabricated, assembled and packaged to deliver a working prototype, that will then be demonstrated for use in the gas and FBG sensing applications.Funding AgencyHorizon Europe
-
TitleA-DASA: Adaptive Mixed-Critical Distributed Architectures For Space ApplicationsSummarySatellite technologies have become more appealing through lower-cost access to the space enabled by innovations like reusable rockets. Constellations of nanosatellites offer a cost-effective way for utilizing a wide range of sensors in space for new research into climate modelling, agriculture, transportation, and others. The onboard computing systems on these nanosatellites process large volumes of sensor data and manage its largely autonomous operation, requiring timely processing and strict deadline-driven activations. The ability to alter their compute capabilities can further the life of nanosatellites by repurposing the older and/or lower reliable systems for alternative lower critical tasks. Hybrid Reconfigurable SoCs are an ideal platform for such applications offering flexibility, post-deployment configurability at hardware and custom processing and interfacing capabilities. Adaptive applications like Software Defined Networks have leveraged FPGAs to provide extra flexibility by enabling the hardware architecture to be modified (through dynamic reconfiguration), on top of the existing software flexibility. However, most research into using FPGAs for self-contained adaptive systems use an ad-hoc approach for coupling software and hardware elements, limiting their usage to experts and specialized tool flows. In this research, we aim to develop a computing infrastructure that will empower application developers to build and deploy applications on hybrid FPGAs in nanosatellites without worrying about low-level hardware details.Funding AgencyHEA
-
TitleAI and Process Automation for Sustainable Entertainment and MediaSummaryEMERALD is a 30-month IA to develop and demonstrate exemplary tools for the digital entertainment and media industries using AI Machine Learning and Big Data technologies, to automate and speed processing, increase production efficiency, use less energy and increase the quality of content. There is a massive increase in the volume of video-based and extended reality content, with an unsustainable demand for skilled human resources, data processing and energy. EMERALD aims to meet the challenge by developing process automation for sustainable media creation; creating a testbed for measuring the energy used in media computation; developing more efficient data use for AI/ML in entertainment and media applications; reducing the power demands for large-scale media data processing; and creating acceptance and demand for AI and sustainable production technologies in the entertainment and media industries. The interdisciplinary Consortium of seven partners includes leading companies from the movie, broadcast, streaming and live entertainment technology sectors, supported by two major European universities.Funding AgencyHORIZON-IA
Computer science - high performance computing, hardware and architecture, Computer science - high performance computing, hardware and architecture, Energy Efficiency, Communication engineering and systems; telecommunications, Information & Communication Technology, Communication engineering and systems; telecommunications, Electrical & electronic engineering, Electrical & electronic engineering, Computer science - Artificial Intelligence, Computer science - Artificial Intelligence,
Recognition
- Best Paper Award - IEEE COINS, 2022 for our paper titled "FPGA-based Deep-Learning Accelerators for Energy Efficient Motor Imagery EEG classification" 2022
- Member, Association for Computing Machinery
- Reviewer and TPC member for International Conferences on FPT, FPL, DATE, ASD and ANCS Conferences.
- Reviewer for IEEE (TVT, TCAS, OJCAS), Springer CSSP journals